There is no doubt that IoT has been incorporated in nearly all aspects of contemporary lifestyle, with the application of this technology being investigated in multiple areas, from hospitals, cities and industries to homes, agriculture, and vehicles. In order to produce optimal, customized and smart decisions, IoT has been further enhanced with intelligence via Machine Learning (ML). Traditionally, collaborative ML methods followed a centralised approach, where a central cloud application was fed data from the various participants and trained a ML model able to produce intelligent decisions, such as predictions, detection of threats and efficient allocation of resources. However, such training techniques present multiple limitations, one of the most important being the possibility of data confidentiality, integrity, and availability breaches, since data would flow unencrypted or with minimal protection methods to the central entity. This major drawback of traditional ML in the era of GDPR, has led to the investigation of new paradigms, focusing on the enhancement of data privacy. In addition, the recent shift from a centralised cloud model to an edge-oriented one has introduced a lot of benefits, such as communication efficiency and much faster response time, since in the cloud paradigm data would have to be constantly delivered to the cloud, consuming resources in the meantime.
The TERMINET project embeds intelligence on the edge, by introducing Federated Learning (FL), which is a novel, privacy-boosted collaborative model training process where data are not required to travel to the cloud, but instead remain local to the edge/near-edge; this supports the rapid production of decisions, due to the edge being closer to the point of interest. To aid that, TERMINET integrates Software Defined Networking (SDN)-enabled virtualized Multi-access Edge Computing (vMEC) approaches to support advanced AI mechanisms based on the FL concept at the edge of the IoT ecosystem. During the FL procedure, each FL participant is responsible for locally training a Neural Network (NN) with its own data, and then forwarding the model’s weights to the central entity, responsible for the orchestration of the entire FL procedure, which in turn, aggregates the weights from all the FL participants and produces a global model, able to respond to the needs of all of the participants; the global model is then pushed back to the FL participants, for inference.
As a result, by incorporating such innovative technologies, TERMINET brings intelligence closer to the user, while supporting rapid response time and optimized decisions, and allowing the secure and private collaborative model creation.
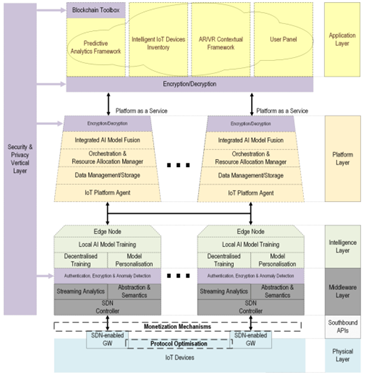
TERMINET’s architecture